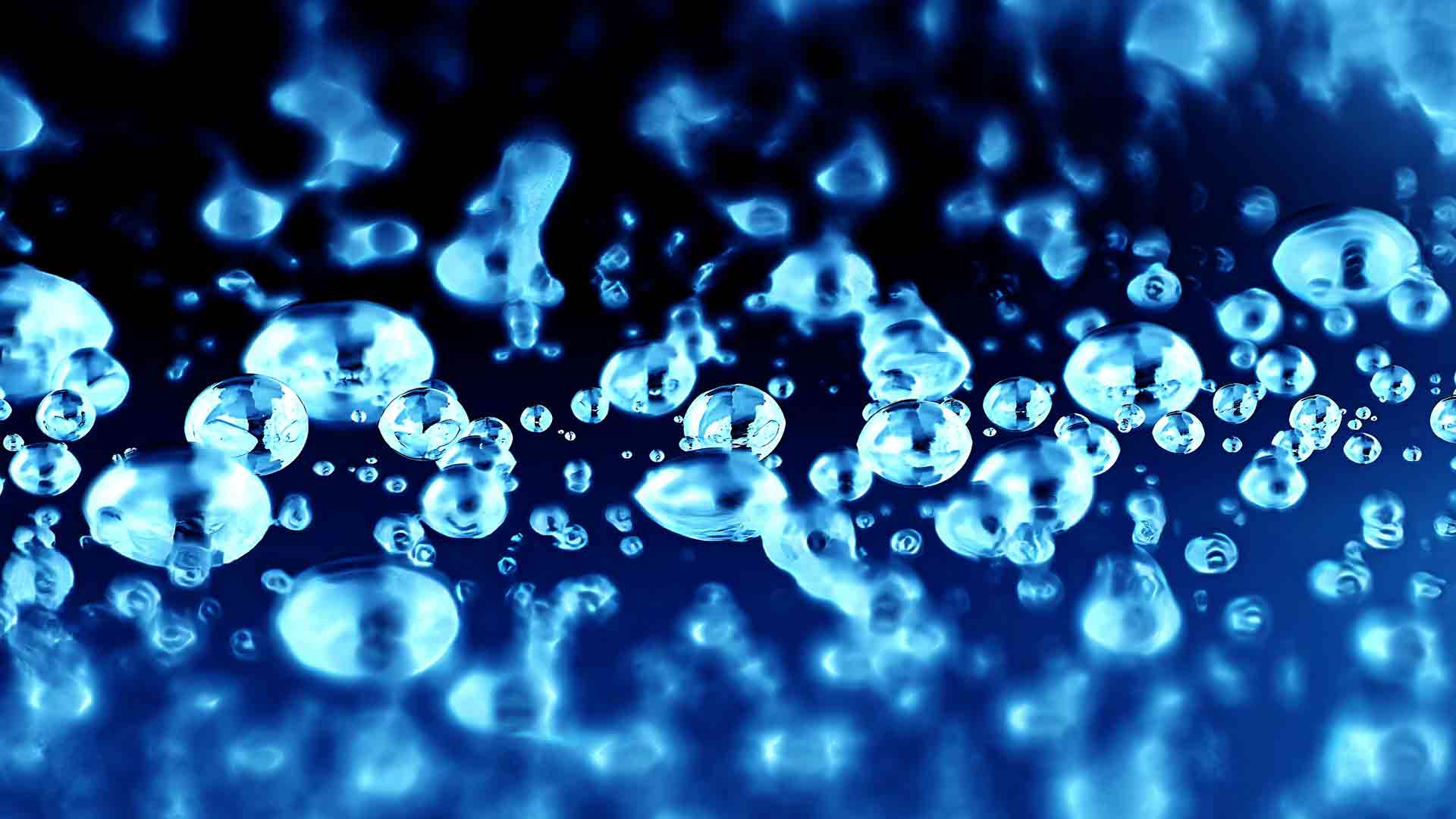
Drug Discovery
How water could replace some organic solvents and make chemical reactions greener
Water as a solvent is more efficient and environmentally friendly.
Read the reportRead the articleDownload the summarySee the infographicRead the publicationRead the recapWatch the video