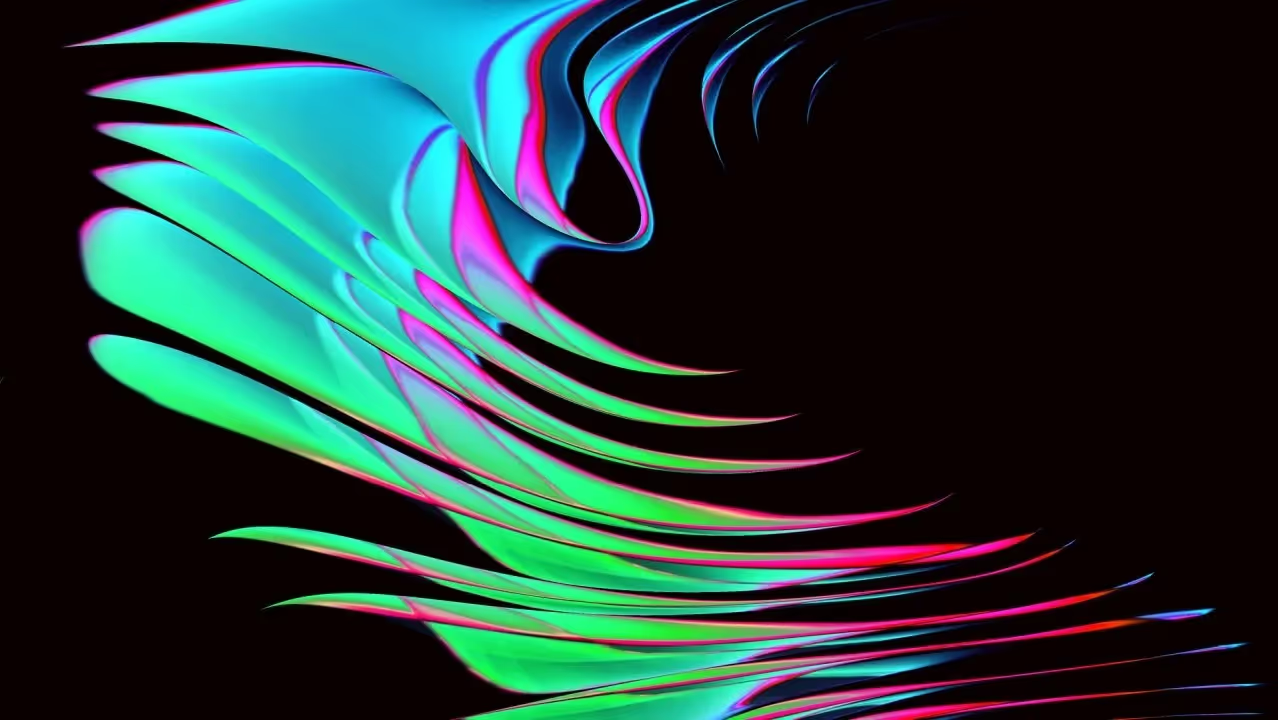
Drug discovery is an ever-evolving field of research, branching out into numerous novel routes such as immunotherapies and gene therapy. At this forefront of development, scientists face a host of drug discovery challenges that hinder progress. It is vital that the industry takes steps to reduce costs and increase discovery efficacy for more financially and medically successful research in the future.
Challenges such as rising costs, modeling limitations, and misunderstood underlying disease mechanisms stand in the way of more effective drug discovery. As research pushes forward into new modeling technologies, we can ask: how is the industry attempting to tackle these challenges? Are the current efforts successful?
The drug discovery landscape
Drug discovery is a broad area of research encompassing many different disciplines. Identifying new drug candidates often requires teams of interdisciplinary researchers to pool their expertise and approach the problem from multiple angles. When we think about the challenges of drug discovery, we are identifying challenges faced by many different key players. Moreover, the therapeutics landscape is now so diverse that these key players differ depending on the type of drug being developed, as each class requires specific expertise.
Challenges can trickle down to all the industries involved with drug discovery and affect the entire landscape, making it a wide-reaching problem with many implications.
Combating rising costs
Drug discovery is a risky investment. It is estimated that fewer than 14% of all drug candidates entering phase 1 clinical trials (the first human trial) gain FDA approval, and the successful ones take, on average, 10-15 years and a $2.5 billion investment to get there. Research and development spending has increased without the number of approved drugs increasing at the same rate, meaning that higher investment per approved drug is needed each year.

With growing investment risks, increasing the likelihood that a drug candidate will be profitable is critical. To achieve this, multiple factors must be considered:
- Poor understanding of the underlying disease mechanisms for which the drug is designed
- Inaccuracy of disease modeling technologies
- Unsuccessful translation of model results to patients
Financial drug discovery challenges can be tackled using a multipronged approach to address all these factors simultaneously. Ideally, the industry can increase the efficacy of its modeling technologies, which can provide a better in-depth understanding of underlying disease mechanisms and more accurate identification of potential drug targets.
Moving on from animal models
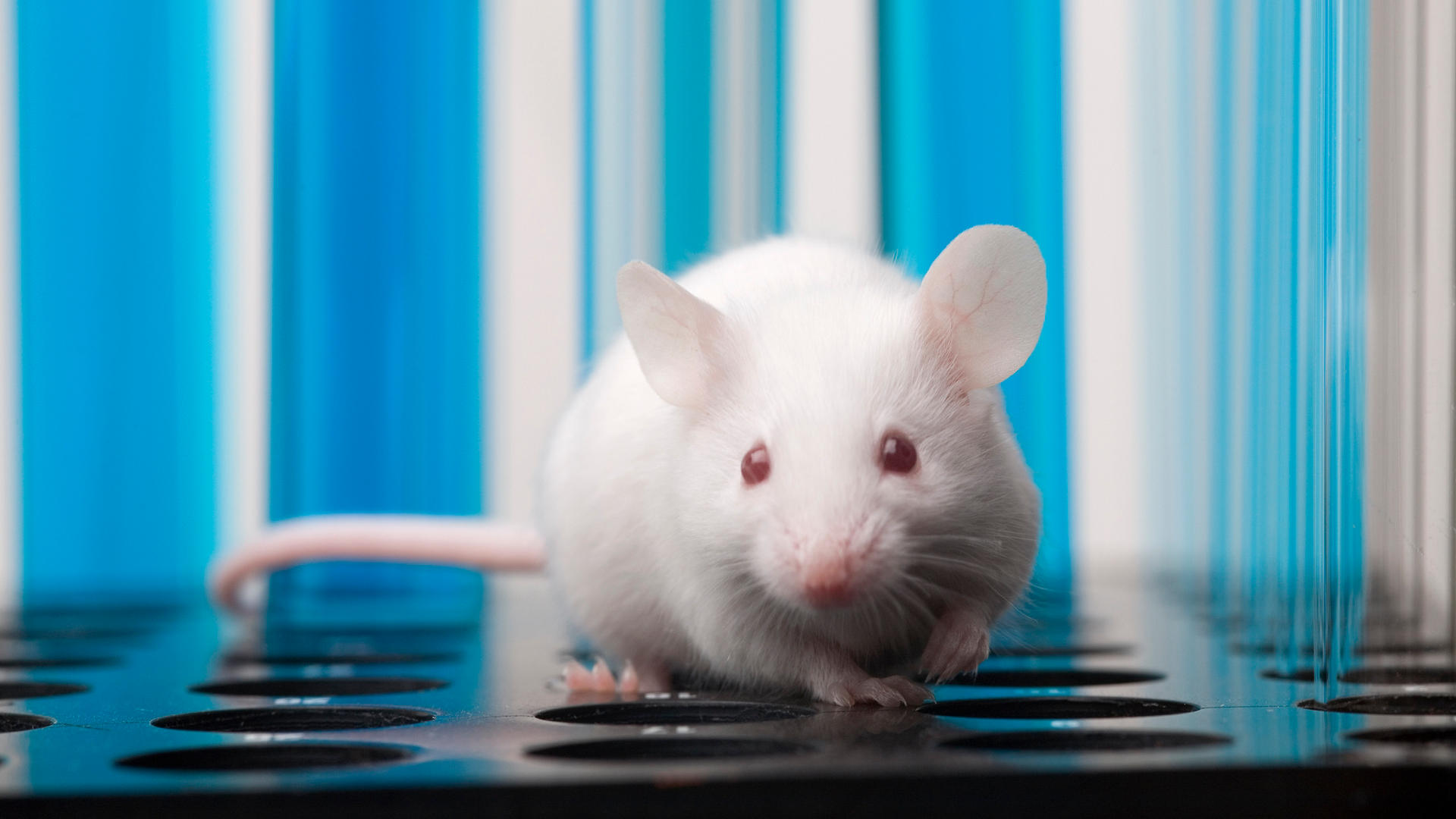
A way to address modeling limitations and poorly understood underlying disease mechanisms is to confront the issues with animal models. Though animal studies have been state-of-the-art in predicting human toxicity during drug discovery and development for many decades, animal models are not without their drawbacks. These include:
- Rarely accurate predictions of human responses
- Increased research duration and cost owing to animal handling
- Negative ethical implications
Of particular concern is the ability of animal models to predict human responses to drugs. While their accuracy has long been assumed, recent studies suggest that this is not the case. This brings about two main problems for the drug discovery process:
- Drug candidates can be incorrectly identified as safe for humans and approved for clinical trials.
- Drug candidates can be incorrectly identified as unsafe for humans and disqualified from clinical trials.
Both of these situations lead to wasted time, money, and resources.
Harnessing the power of iPSCs

One key advancement in modeling for drug discovery is induced pluripotent stem cells (iPSCs). In this technology, pluripotent stem cells are differentiated into cells that model diseases, which can be used to recreate the disease phenotype in vitro accurately. Because iPSCs can be developed as human disease models, they can be used to aid drug development in the areas where animal models fall short.
The ability of iPSCs to mirror the molecular and cellular phenotypes in patients allows for potentially more accurate drug target identification and may be better able to predict a drug’s safety profile in humans than in animal models. Without the hurdle of interspecies comparisons, one of the major challenges of drug discovery, iPSCs are theoretically less likely to falsely identify a drug target, decreasing the likelihood of advancing a lead candidate that will fail in clinical development. Moreover, they should provide a better readout of potential safety liabilities on a molecular and cellular level than tests performed on animal cells or even immortalized human cell lines. While these theoretical benefits of the iPSC technology are yet to be experimentally demonstrated, early analysis of published data suggests that iPSCs have a greater capacity for accurate disease modeling than animal models.
Using iPSCs also helps increase the understanding of disease mechanisms. Since the disease can be modeled more accurately in an iPSC than in an animal, far more of the complex biological picture can be captured. In this way, the use of iPSCs can tackle both the low specificity of animal models and the need for understanding disease mechanisms. Using this kind of modeling alongside traditional methods could reduce the high number of unsuccessful drug targets, thereby reducing developmental costs.
Applying artificial intelligence

Computer modeling has long helped to overcome the challenges of drug discovery in the form of computational chemistry and bioinformatics, but advances in AI can now take this to new heights. With the recent rise in AI popularity, the pharmaceutical development sector has been asking how it can also benefit from this powerful tool, which led to growth in AI drug discovery.
Several companies now offer AI platforms and pipelines for a range of drug discovery needs, including:
ExscientiaApplying AI to small molecule drug discoveryRecursionUsing AI to gain insights into cellular behaviors, including phenomics and orthogonomicsInsitroMachine learning on population scale data and cell-based disease models to provide insights into diseaseValo HealthApplying AI to patient-based dataCellarityUsing AI to view a disease across the whole cell rather than a single targetAbCelleraApplying AI to antibody-drug discoveryXtalPiAI for small molecule and macromolecular drug discovery.AtomwiseApplying AI to small molecule drug discovery.SchrödingerAI for small molecule and biologics drug discovery.
Leveraging the power of these technological advances can enable companies to cut costs and increase the chances of drug candidates gaining successful approval. The German biotechnology company Evotec followed this path and, in 2021, announced the entry of their immuno-oncology drug, discovered in collaboration with Exscientia, into human clinical trials. Collaborations of this kind and the integration of AI into existing pipelines can forge a path for future drug discovery.
Are we dealing with the challenges of drug discovery?

With research tackling the challenges of traditional animal modeling with newer AI and iSPC technologies, great strides are being made toward eliminating wasted time and resources and increasing the chances of drug target success. Since both of these technologies decrease research costs and increase the likelihood of a drug candidate’s success, we will likely see their impact closing the financial gap between drug discovery expenses and approved drugs in the coming decade.
Companies in the industry should make a concerted effort to integrate these new technologies with their existing drug discovery pipelines to keep pace with their competitors as the future of the industry begins to take shape.